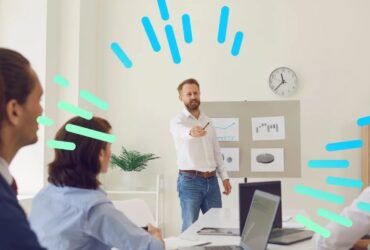
Insights
Considered purchases are a significant hurdle across many different industries. The nature of purchases with longer conversion paths crosses over from the likes of wedding dresses, vehicles, kitchens or holidays, there is a common challenge that behavior modeling can help streamline.
Utilizing behavioral modeling allows you to introduce individuality to each user and create a probabilistic outcome for each individual user’s behaviors and attributes as they browse online.
Adopting this approach of attributing behaviors moves away from the traditional bottom-of-the-funnel attribution models focused on a number of leads and instead measures the quality and predictability of user’s behaviors to combat the challenge of long-form, considered purchases.
Most considered purchases of a sizeable value require multiple visits from a customer before a decision is made. It is very common that this decision is not made in isolation and may involve other stakeholders, such as a partner, which makes for a tracking nightmare.
You will almost certainly find the process extends beyond the lifetime of a cookie and likely transpires over multiple people using multiple devices. It’s clear when you take a step back that no conventional approach to attribution modeling, such as switching to first-click attribution, will solve this issue.
Getting your CRM data into a place where it can be accessed for modeling is key to centralizing your data. We use Google BigQuery and follow a process that enables us to join online activity through Google Analytics (GA) with all the offline data held within a CRM.
Taking this a step further, we implement a soft login solution to the website that enables us to maintain a user’s ID each time they provide identifiable information. For example, a user who requests a brochure will be assigned an ID within the CRM. This same ID is then used each time they identify themselves on the website so that GA can better join the dots and we are less reliant on all actions occurring within the cookie window of a singular device.
Rather than attributing the success of a sale back to a holiday brochure six months ago that has long since passed, it’s about transforming your business to predict the future success of today’s user based on their individual behaviors.
Centralizing and automating your data comes first with the potential to accomplish a lot with a singular extract of CRM data. In the future, you can look at harnessing machine learning to dynamically value each individual lead and pass that value back into Google Analytics for optimization.
The potential within automation and machine learning can be daunting, which is why it’s important to start small and gradually evolve your strategy.
Estimated value modeling also enables you to fill in the blanks where user journeys are broken. Since we are valuing behaviors themselves, that behavior occurs regardless of if we can tie that individual back to a sale later down the line and we can use historic data to model the value of those behaviors based on other user journeys that have been joined up. This circumvents issues seen with most attribution models where that user would instead have no value at all.
Since GA data is now involved, this includes a vast wealth of data that occurs prior to a sale. This data opens the door beyond bidding and allows you to implement estimated value modeling (EV) to all touchpoints based on user behavior. This includes valuing every session individually and incrementally increasing or decreasing the value of a user’s sessions as we gather new information on that user.
It then becomes possible to run prospecting optimized towards an ROI target. You’re no longer running prospecting and presenting the results in form fills and transactions, it’s about understanding the value of each session and optimizing towards the quality of those sessions based on their predicted future value to the business.
Many businesses are focused on building an econometric model based on cookies when really, they should be focusing on tying up collected info throughout the user journey and attributing to those points.
Scoring individual users allows you to build an intimate understanding of your audience and begin segmenting customers based on value. The audiences can be implemented across your digital platforms to transform your remarketing strategy into one based on an individual user’s value.
By predicting the future value of every touchpoint that occurs across your online and offline environment, you’re able to make marketing decisions today that drive future growth. This process moves away from attempting to optimize today’s performance when the customers who bought today did so because of touchpoints they had two months ago that are already out of your control.
It’s important to select inputs that are most likely to correlate with an increased or decreased chance of a user purchasing. For example, the type of brochure downloaded, page paths taken through the website and number of visits to the site.
These inputs are then built into a machine-learning model that determines the likelihood of conversion based on every possible permutation of these inputs and the historic outcome of those sequences.
Once built, these models are tested for their accuracy and an iterative process occurs whereby we tweak the model to improve its predictive capabilities. These models run daily and continue to evolve over time as they gather new data and react to new trends.
The output of them is typically pushed into GA for use as digital channel KPIs, but they also open up substantial opportunities with business intelligence to analyze the business pipeline offline as well.
Most importantly, a user’s journey does not end with a sale. An estimated value model continues to assess the value of a customer after they have purchased. Their value will decay over time, as does the value of all touchpoints in an estimated value model, but any new touchpoint where this customer has identified themselves will be evaluated using the knowledge that they are an existing customer.
If considered purchases are a problem for your business and you would like to know more about predictive modeling, we go into more detail on the subject in our latest whitepaper.
Other topics covered in this whitepaper include:
Insights
Insights
Insights