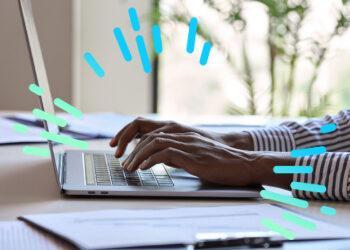
Insights
It seems everywhere you look these days, there is talk of AI taking over. We have already reaped the rewards of machine learning in many areas of digital marketing – for example, Google Ads’ smart-bidding uses machine learning algorithms to adjust bids in real-time to help campaigns achieve their targets, while data-driven attribution uses machine learning to decide how much credit to give each touchpoint in a user journey.
Machine learning is used in organic search, too; Google itself uses multiple machine learning algorithms to assess and rank websites (RankBrain, BERT, etc), and here at Search Laboratory we have built multiple tools which use machine learning to help us assess and improve various factors in organic search. One area which has gained a lot of interest over the last few years is the use of machine learning in content creation.
Content is a core element of any digital marketing strategy, and it is used across all stages of the customer journey to answer user queries and push them along the sales funnel. When it comes to SEO, having quality content helps to determine whether you appear on page one in the SERPs – and with 71% of organic clicks going to the first four results, appearing in those top positions is crucial for generating traffic and sales.
From researching keywords, to creating a content strategy, to delivering a variety of content pieces, producing high-quality content is time-consuming. One of the biggest benefits of machine learning is that it dramatically cuts down the amount of time required to do a job, and when time means money, this is a very attractive benefit.
While the thought of robots writing content may seem far off in the future, many publishers already use machine learning to create content – for example, the Associated Press use machine learning known as natural language generation to sift through data and write sports reports.
Natural language generation (NLG) is a software process that turns data into a written narrative within seconds. NLG requires data to be cleaned and structured before being uploaded into an NLG system. You will also need to define the formats for different types of content through templates and rules – the structured data is then fed into and formatted by these rules to produce data-driven narrative content.
Most publishers who use NLG to produce content will still have a human proof the pieces before they go live, but the hours saved (which can be in the thousands for those who produce hundreds of articles) frees up the teams to focus on strategy and editorial.
Natural language generation is adept at producing data-driven narratives at great speed, which at first glance seems like a winning solution for SEO teams.
However, when you look deeper there are many issues. For one, the time and effort required to clean and structure data can be immense, and it will need to be carried out by data scientists – and their time is likely best spent elsewhere! For another, it relies on the need for data in the first place. Many users conducting searches are looking for qualitative answers which will help them to decide what solution they need for a particular problem – a data-driven and number-focused report is unlikely to help them find an answer.
Search engines aim to deliver the best and most useful content to users. As much as machine learning algorithms like NLG can understand sentence structure and grammar and how to write words around data, how good are they at invoking emotion? Do they really understand customer pain points and how to overcome them? Can they express opinion and brand tone of voice? Do they have the expertise, authority and trustworthiness – the three key components in Google’s E.A.T concept, which is used to assess and rank content?
A large proportion of content which is created in line with SEO objectives are not data-driven narratives, they are creative solutions to search queries – something humans are better at than machines.
There is no doubt that if you want to produce quality content, the best person to do it is in fact a person. However, that does not mean machine learning must be avoided at all costs. While creativity is generally best left to humans, there are tasks which can be automated to improve efficiency and results, including:
Using machine learning and automation in this way is known as content intelligence, and the insights provided can be used to create high-quality, audience-targeted content which yields better results.
Examples of content intelligence tools include Buzzsumo, Concured and Ceralytics. We have also built our own content intelligence tool, the Content Relevancy Checker, which uses machine learning to determine how relevant search engines will determine a webpage is to the keywords it is trying to rank for.
Organic search is evolving very fast in today’s digital landscape. Generative AI is now part of search engine results, user journeys now move across multiple channels and Google’s continued core updates are reasons for shifts in SEO habits worldwide.
The Future of SEO report, co-authored by James Bentham and Amy Banks, addresses these concerns and provides insights for marketers enabling them to realign their approach to SEO.
The result is that marketers can develop future-proofed SEO solutions for businesses that lead to long-term success.
Insights
Insights
Insights